DeepSeek R1 Open Source Models Selecting the Right Architecture with RAG
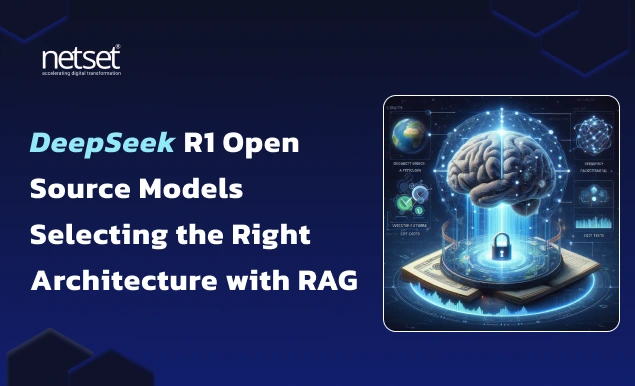
We did not think that artificial intelligence would achieve remarkable efficiency and reshape nearly every industry, that too in a very short period of time. Just look at OpenAI; its models have set a gold standard in the space of AI because they are so good at reasoning and their generative capabilities.
But, yes, the cost of training these models is mostly high and may give you limited flexibility, which is why there is one more player in the market achieving wonders with its open-source model—DeepSeek R1. You get high-quality reasoning and retrieval potential but without breaking your pocket or bank.
If you are a business seeking AI development services for Retrieval Augmented Generation, or RAG, then you must know how to select the right architecture. Here is a detailed guide for you.
A Brief About DeepSeek R1
DeepSeek R1 is a modern open-source AI model built by DeepSeek AI in China. When comparing other preoperative models like the ones from OpenAI, DeepSeek R1 gives a much more budget-friendly and highly custom solution for building AI solutions.
Its powerful reasoning and retrieval capabilities stay as the key strengths, which is why it is becoming an excellent choice for a wide range of apps that want to integrate natural language processing and data analysis.
The development of DeepSeek R1 was inspired by the principle of keeping it affordable but the most powerful AI model so that it could compete with the leading solutions.
Further, the company made this model open source which gives strength to developers and businesses in getting better technology and that too at lower cost.
Some of the key features of DeepSeek R1 are:
- Great reasoning and retrieval: As R1 excels in providing accurate and context-aware responses due to its advanced architecture.
- Flexible: It is an open-source model that you can modify or customize according to your business needs and requirements.
- Community Support: The open source nature of DeepSeek R1 inspired a lot of developers to collaborate and innovate in the AI space.
Businesses who were looking to hire ChatGPT developers, are not seeking DeepSeek developers because of its accessibility and adaptability. In the near future, DeepSeek may take the top place in the AI market.
How are open-source models better than any other model?
There is one common key question that will come to anyone’s mind is, “why open source AI models are in so much hype?” Let us answer this.
Since AI is still in its developing phase (for now), open-source AI models play a key role in raising this field as they provide a range of advantages over commercial models.
Such models (like DeepSeek R1) are available for free and developers can use, modify, and even distribute it as per their requirements. This freedom creates an environment of collaboration and innovation in the space of AI.
Free yet boosts innovation
The one primary benefit of open-source AI models is their cost-effective nature. When a model is Proprietary it requires expensive licensing and subscriptions which makes them less accessible to a lot of developers and startups who are low on budget. But, when a model is open source, like the DeepSeek R1, then developers and businesses have technologies to work on, without any cost.
Flexible and Customizable
The next benefit of open-source AI models is flexibility because developers can customize these models to meet the specific needs and requirements of businesses as well as their users. Just like Linux is sold in multiple flavors, the same goes for the AI models. Proprietary models were very strict and less adaptable.
For anyone wanting to engage in AI development services, open-source models like DeepSeek R1 are the best and most versatile choice for them. You get all the mentioned benefits with open-source models.
The Concept of Retrieval Augmented Generation
Now coming to the RAG concept, by now you must be wondering to explore more about Retrieval Augmented Generation. Well, it is a powerful technique that boosts the ability of AI to generate correct and responses that highly aligns with context. It makes use of the power of retrieval based and generative approaches, thus making a hybrid model that gives the best of both worlds.
When it comes to a retrieval-based approach, the AI searches a database or knowledge base to look for the relevant information on the basis of the input query. Just because of this process the response stays reliable in factual terms as well as it aligns with the context of the query.
The generative approach involves AI using its internal model for creating a response from scratch, which sometimes misses creativity and flexibility in generating the answer.
RAG mixes both approaches! When a query is received, the AI first fetches some relevant information from a pre existing database. This fetched data is then sent into the generative model, which further uses it to create an accurate and context related response.
Also, the benefits of RAG are very evident especially in the apps that are of complex nature consisting of deep natural language processing, customer support, and research. For example, ChatGPT developers or AI development service providers can use RAG to build AI systems that can provide the most accurate answers to the queries of users even when a topic is technical in nature.
When a business incorporates RAG into AI models like the DeepSeek R1, it can deliver some notable enhancements in the performance.
Selecting the Right Architecture for DeepSeek R1 with RAG
For anyone who wants an optimized performance and wants to achieve desired outcomes, then choosing the right architecture becomes important. There are several factors that go into architecture selection, like task requirements, performance, scalability, cost, and a lot more.
Know your requirements
Start the process only after understanding the requirements. It is because different tasks (like natural language processing and computer vision) require different types of architecture. For example, if you are working with NLP, it might be using transformer models. These models are known for their potential of handling big amounts of text based data and generate quite relevant answers to users.
Focus on performance and scalability
Do not forget about performance and scalability! The architecture you should have shall be capable of handling the expected workload and scaling up as you need it to be in the future.
Transformer models are a great fit for this kind of high-performance application because of their parallel processing capabilities and ability to manage big datasets.
Further, make sure to keep your focus on the cost and resources which plays an important role in architecture. Well, there are some architectures which give great performance, but they may also require good computational resources and other infrastructure investments.
You have to balance performance with cost-effectiveness to make sure that your AI projects stay sustainable. These open-source models, like DeepSeek R1, give a cost-effective option to other solutions.
Common architectures with DeepSeek R1
- Transformer Models
- Sequence to Sequence models
- Hybrid models
Few Implementations and Best Practices
To implement DeepSeek R1 with RAG successfully, you must know the key implementation steps and some best practices.
Here are the steps:
- Start by setting up and configuring the DeepSeek R1. The official documentation is the best place to learn about DeepSeek AI.
- Do make sure that all the dependencies are installed and the model is accurately connected to your development environment.
- Apply RAG with DeepSeek R1 by creating a database or knowledge base that has relevant information for fetching.
- Make configuration of the fetching components to search this database on the basis of queries.
- Once you have set up the fetching, start feeding the fetched data into a generative model to show responses to the end user.
Some of the optimization best practices include:
- Regularly updating your database with new and relevant information.
- Fine-tuning the generative model based on specific use cases and feedback.
- Monitoring and evaluating the system’s performance to find out and work on any bottlenecks or issues.
Boost Your AI Projects with Our DeepSeek Expertise
If you are looking to take your AI project to the next level or start something from scratch, NetSet Software can help you. We are one of the most reliable AI development service providers, and our team always adds the most advanced technologies, whether it is OpenAI or DeepSeek.
At very cost-effective prices, we can build an AI application for you that not only promises innovation but also promises the required attention from your targeted users. Book a call with us right from our Contact Us page and complete your AI product vision.