Understanding The Role Of Artificial Intelligence in Developing Robust and Responsive Supply Chains
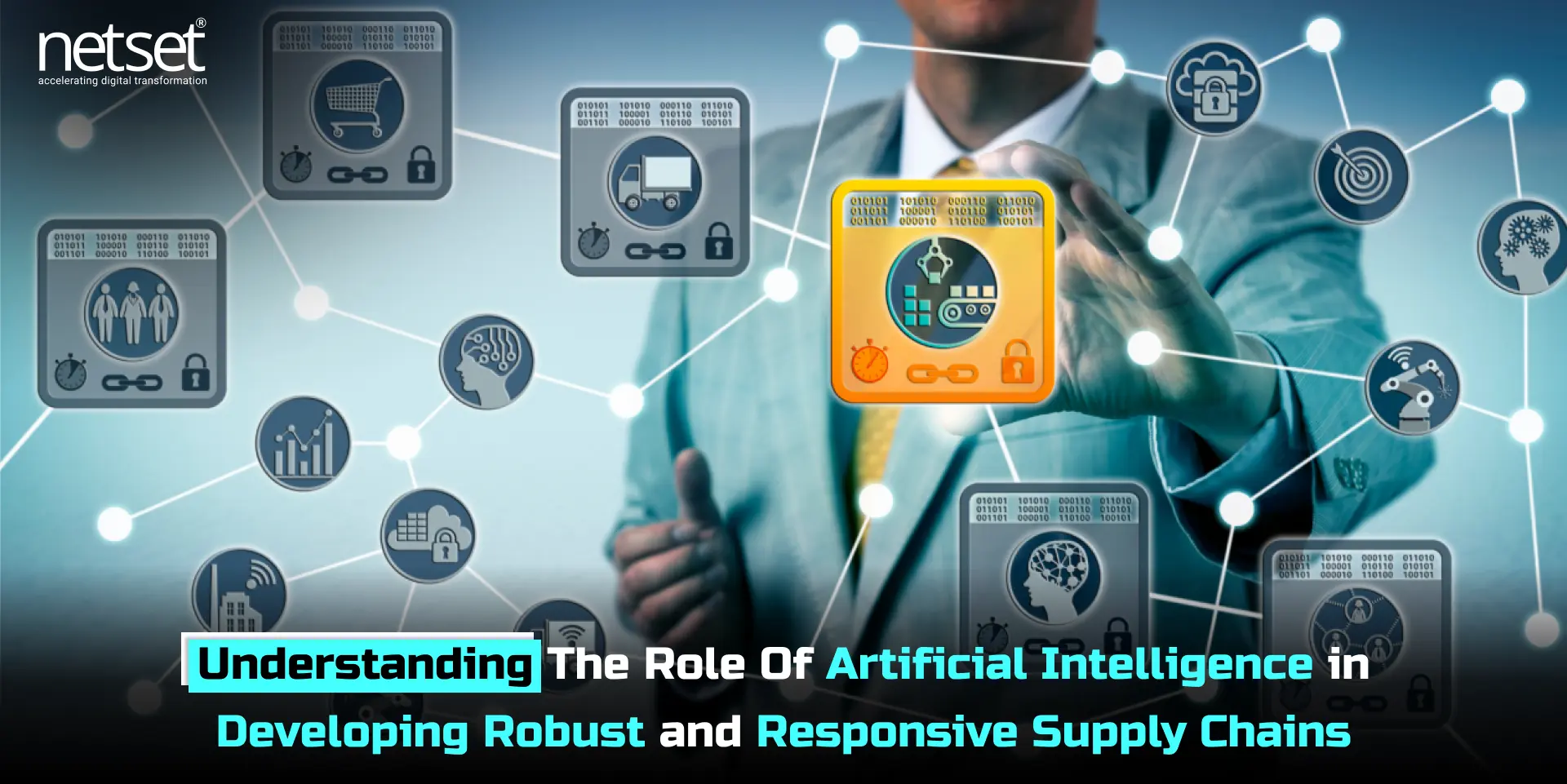
Many industries still face challenges following the pandemic. However, others, such as supply chain, are embracing new technologies, including Artificial Intelligence (AI) and Data Analytics, on a large scale. These innovative technologies transform supply networks into robust, efficient, and adaptable.
Recently, supply chain and logistics sectors have begun to unlock the potential of AI, from optimizing truck loading to forecasting demands. Machine learning stands to transform these industries fundamentally. But what’s the mechanism? How exactly does AI facilitate the physical movement of goods from origin to destination?
This blog will guide how AI and data analytics play an essential part in improving supply chain management. We will also go through the steps that are required for optimizing these technologies, and discuss the use cases for mitigating disruptions via AI capabilities. You will also learn how enterprises can benefit from and invest in AI software development solutions.
What Is The Role Of Data Analytics in Supply Chain Management?
AI-driven data analytics converts unprocessed and raw data into meaningful insights and information. This valuable information is crucial for supply chain management. The contributions of various forms of analytics are as follows:
Predictive analytics: This kind uses past data to project future events, which helps with managing inventory levels, predicting demand, and averting possible disruptions. Predictive analytics can be used, for instance, by a business to stock up on popular items ahead of peak season.
Descriptive analytics: It helps firms understand what went wrong and why by giving them a quick overview of both past and present operations. Analyzing delivery times across several geographies to spot bottlenecks is one example.
Prescriptive analytics: Based on both descriptive and predictive analytics, this approach recommends actions that are going to take place in the coming time. For instance, this analytics can suggest most efficient shipping routes by evaluating multiple factors like cost, time, carbon impact, and more.
Cognitive Analytics: By processing unstructured data, such as social media sentiment, cognitive analytics can get deeper insights into customer behavior by using artificial intelligence (AI) to simulate human brain processes. One such would be modifying supply chain tactics in response to immediate customer input.
How To Optimize AI and Data Analytics in the Supply Chain for Improved Efficiency?
AI development services and solutions have transformed supply chain management, offering exceptional opportunities for efficiency and innovation. While the process for fully incorporating these technologies can vary depending on each organization’s individual needs, resources and industry landscape. There are universally effective steps which have been outlined by Netset Software as one approach:
Crafting a Blueprint: Establishing Strategic Goals
First and foremost, you need to define what your desired outcomes for AI/analytics integration into supply chain operations are. Netset Software begins this process by consulting with stakeholders on which areas they believe AI could make an impactful difference such as improving demand forecasting, optimizing inventory levels, route planning enhancement or risk management strategies.
Gathering and Structuring Data as the Base for AI
Collect relevant data across your supply chain – this includes sales figures, customer interactions, inventory records, logistical details, and even external factors like market trends or weather forecasts – and organize it effectively for effective analysis.
Preparing Accurate and Consistent Data
Raw data contains inaccuracies, inconsistencies, and missing elements that need to be cleaned up prior to using AI technologies. Cleansing involves eliminating duplicates, correcting errors, and filling any gaps that arise in its layout, and formatting of this dataset correctly.
Algorithm Selection: Tailoring AI to Your Needs
Assembling the right AI algorithms is key to meeting specific challenges within your supply chain. From regression analysis and classification techniques to clustering methods or deep learning for complex pattern recognition, finding an algorithm tailored precisely to meet each task at hand is of critical importance.
Technology Selection: Selecting Appropriate Tools
Once you understand your objectives and data sources, selecting appropriate AI technologies should be easy. This may involve applying machine learning, predictive analytics, robotic process automation, computer vision or natural language processing tools that support your goals.
Data Modeling: Formulate an Artificial Intelligence Framework
Data modeling involves selecting and fine-tuning machine learning algorithms to best interpret your data. This step involves experimentation and feature development, so that chosen algorithms accurately predict and analyze supply chain dynamics.
Integration: Integrating AI With Existing Systems
Integrating AI capabilities into your existing supply chain infrastructure is of utmost importance, whether that means linking AI models with enterprise resource planning (ERP), warehouse management systems (WMS), transportation management systems (TMS), or any other applicable platforms to ensure seamless data flow and operation.
Testing and Validation: Ensuring Reliability
Testing and validation are an integral component of AI integration. At this crucial juncture, AI models and integrated systems undergo rigorous trials to ensure they not only perform as intended but also enhance supply chain operations without disruptions. This step includes conducting simulation tests with real world outcomes to stress test AI algorithms for precision, reliability, and overall performance evaluation.
Continuous Evolution: Support Ongoing Optimization
AI-enhanced supply chain management does not end with its deployment; rather, it involves an ongoing cycle of evaluation, refinement, and adaptation. Netset Software actively encourages businesses to follow this philosophy by regularly evaluating AI solutions’ performance and impact on their supply chain processes. Being sensitive and responsive to new data sources, technological innovations, and market requirements allows businesses to ensure their supply chains not only meet current challenges but are future-proofed against tomorrow’s uncertainties.
Real-World Use Cases Of AI Development Solutions For Controlling Supply Chain Disruption
Supply chain disruptions in today’s global market are not just a problem, but a fact that companies face. AI companies offer innovative Artificial intelligence development solutions that can mitigate this problem, and improve visibility, predictability, and efficiency. Here are five key use cases where understanding and analytics are making significant advances in managing supply chain disruptions:
Improved demand forecasting: Intelligent predictive analytics is changing the way businesses predict customer demand. By analyzing large data sets, including historical sales data, market trends, consumer behavior, and even social media sentiment, AI algorithms can predict demand with precision right This view allows companies to adjust production plans, inventory levels and distribution plans, thereby significantly reducing the risk of overruns or overruns and ensuring better supply chain operations. .
Smart inventory management: Smart intelligence and analytics change inventory levels based on real-time demand forecasts and supply chain processes to improve inventory management. This approach minimizes the costs of a large amount of savings or reductions. Machine learning models can take into account factors such as lead time, supplier reliability, and historical demand patterns to identify patterns and predict optimal levels for different locations. This knowledge allows products to be available when and where they are needed, reducing the impact of supply chain disruptions.
Predictive maintenance: In parts and vehicles, equipment shortages can cause major problems in the supply chain. AI-enabled Predictive Maintenance uses data from IoT sensors to predict when to perform maintenance to prevent failure. Artificial intelligence models, by analyzing historical records of maintenance and real-time information on equipment condition can predict potential failures and enable timely maintenance.
Supplier risk management: Insights and analysis provide high-level capabilities to assess and mitigate supplier-related risks. By assessing data on supplier performance, financial stability, geopolitical risks, and even environmental conditions, AI models can identify risks that can be anticipated and planned. reduction strategy. This dynamic approach allows companies to change their supplier base or develop contingency plans, reduce reliance on a single source and increase supply chain efficiency.
Route optimization and logistics planning: Artificial intelligence algorithms excel at analyzing logistics data in order to optimize transport routes and delivery schedules. AI can find the best routes by taking into account factors such as weather forecasts, traffic conditions and fuel prices. By considering factors such as traffic conditions, weather forecasts, fuel prices and delivery times, AI can identify the most efficient routes. This capability not only saves time and reduces shipping costs, but also reduces the risk of delays and ensures that products reach their destination as planned if problems occur. suddenly
Make Your Supply Chain Future-Ready With Netset Software
Netset Software, an AI and blockchain development company at the forefront of empowering businesses to build resilient, efficient, and adaptable supply chains, with cutting-edge artificial intelligence development services including AI software development solutions and conversational AI software solutions, which transform supply chain operations. Their experience ensures your supply chain operations are prepared for tomorrow while driving efficiency and innovation forward.
FAQs
In what ways does Artificial Intelligence (AI) affect supply chain management?
Supply chains employ artificial intelligence development solutions in many ways. They are able to automate tasks, analyze vast amounts of data and make precise predictions. AI software can help with data analytics, forecasting demand, order processing and risk assessment.
How does AI affect supply chain performance ?
By leveraging AI software development services to automate repetitive tasks, supply chain can achieve increased efficiency and reduce costs. AI software services with predictive capabilities enable better demand forecasting. This leads to improved inventory control. AI is also capable of identifying potential supply chain risks, allowing companies to take proactive measures in order to mitigate those risks.
What is AI’s future in supply chain?
AI will continue its evolution and be expected to create even more profound changes in the supply chains sector. AI development will revolutionize supply chains, from autonomous operations to predictive analytics. AI-powered solutions provided by conversational AI are proving to be highly beneficial throughout the entire supply chain.
How are AI and Machine Learning applied in supply chain Management?
AI and Machine Learning (ML), facilitated through AI development solutions in supply chain management, is used to analyze and make accurate projections from large quantities of data. These solutions can help with areas such a demand forecasting, transportation and inventory management, as well as risk identification. ML algorithms are able to learn from past data and make predictions for the future. Businesses can plan their operations better by using this technology.